STAC Report: Paperspace Cloud with NVIDIA A100 and H100 GPUs under STAC-AI LANG6 (LLM inferencing)
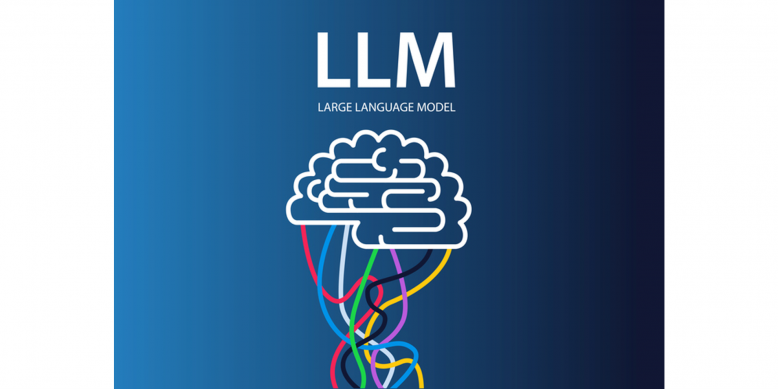
First STAC-AI benchmark results released
20 December 2024
The recently released STAC-AI™ LANG6 (Inference-Only) benchmark sets a new standard for evaluating infrastructure performance of Large Language Model (LLM) applications. Developed in collaboration with AI experts and technologists from leading organizations, it provides a comprehensive assessment of the performance, quality, and resource efficiency of any technology stack powering LLM workloads.
STAC recently performed four STAC-AI™ LANG6 (Inference-Only) audits using virtual machines (VMs) in the Paperspace cloud. STAC undertook this work to provide initial examples of the value of detailed benchmark reporting of the software and hardware stacks used for LLM Inference for realistic financial use cases.
Two Stacks Under Test (SUT) were tested, the first featuring 8 x NVIDIA A100-SXM4-80GB GPUs, and the second featuring 8 x NVIDIA H100-80GB-HBM3 GPUs. For each SUT, separate audits were performed for the Llama-3.1-8B-Instruct and Llama-3.1-70B-Instruct models. Note that STAC is solely responsible for these results: No hardware, software or service vendors participated in these audits.
The STAC-AI™ LANG6 (Inference-Only) benchmark includes both batch and interactive use cases. Interesting results from the batch mode testing include:
- For the Llama-3.1-8B-Instruct model, the SUT featuring the H100 GPU averaged 2.1x the Batch Inference Rate1 and 1.1x the Batch-mode Price Performance2 across the two Data Sets tested compared to the SUT featuring the A100 GPU3.
- For the Llama-3.1-70B-Instruct model, the SUT featuring the H100 GPU averaged 2.4x the Batch Inference Rate4 and 1.3x the Batch-mode Price Performance5 across the two Data Sets tested compared to the SUT featuring the A100 GPU6.
Note that Price Performance results are based on the retail, hourly prices for provisioning the SUTs in the Paperspace cloud.
For interactive use cases, the STAC-AI™ LANG6 (Inference-Only) benchmark is unique in that it relates LLM performance to user satisfaction, or the ability of the LLM output to smoothly feed downstream tasks. Interesting results from testing the SUT featuring the H100 GPU include:
- For Llama-3.1-8B-Instruct, the SUT was able to maintain a 5P Output Profile of 10.7 words per second (WPS) at the maximum arrival rate tested for the EDGAR4a Data Set (44.0 requests per second). This is well above the mean, sustainable human reading rate of 4.0 WPS (with std. dev. +/- 0.85 WPS) for non-fiction observed over many studies.7,8
- For Llama-3.1-8B-Instruct and the EDGAR5a Data Set, the 5P Output Profile (4.98 WPS) at the fastest arrival rate tested (0.394 requests per second) is still above the mean rate cited above.9
- For Llama-3.1-70B-instruct, the SUT was able to maintain a 5P Output Profile of 6.22 words per second (WPS) at the maximum arrival rate tested for the EDGAR4b Data Set (3.33 requests per second). This is also well above the mean rate cited above.
The EDGAR4a/b Data Sets mentioned above model a Retrieval Augmented Generation (RAG) workload based on EDGAR securities filings, having a median initial context size of approximately 1,200 words. The EDGAR5a Data Set represents question-answering against an entire EDGAR 10-K filing with a median initial context size of 44,000 words.
The audit reports are publicly available after a free registration.
https://www.STACresearch.com/STAC240903a (NVIDIA A100, Llama-3.1-8B-Instruct)
https://www.STACresearch.com/STAC240903b (NVIDIA A100, Llama-3.1-70B-Instruct)
https://www.STACresearch.com/STAC241122a (NVIDIA H100, Llama-3.1-8B-Instruct)
https://www.STACresearch.com/STAC241122b (NVIDIA H100, Llama-3.1-70B-Instruct)
Premium subscribers have access to extensive visualizations of all test results, the detailed configuration information for the solutions tested, the code used in this testing, and the ability to run these same benchmarks – as is, or with other models and data sets - in the privacy of their own labs. To learn about subscription options, please contact us.
---------------
1 STAC-AI.LANG6.Llama-3.1-8B.*.BATCH.INF_RATE.v1
2 STAC-AI.LANG6.Llama-3.1-8B.*.BATCH.PRICE_PERF.*.v1
3 See https://STACResearch.com/STAC240903a
4 STAC-AI.LANG6.Llama-3.1-70B.*.BATCH.INF_RATE.v1
5 STAC-AI.LANG6.Llama-3.1-70B.*.BATCH.PRICE_PERF.*.v1
6 See https://STACResearch.com/STAC240903b
7 STAC-AI.LANG6.Llama-3.1-8B.EDGAR4a.INTERACTIVE.OUT_PROF.v1
8 Marc Brysbaert, How many words do we read per minutes? A review and meta-analysis of reading rate, Journal of Memory and Language 109, (2019), https://doi.org/10.1016/j.jml.2019.104047
9 STAC-AI.LANG6.Llama-3.1-8B.EDGAR5a.INTERACTIVE.OUT_PROF.v1
About STAC News
Read the latest about research, events, and other important news from STAC.